Enhancing Object Detection with Image Labeling Tools
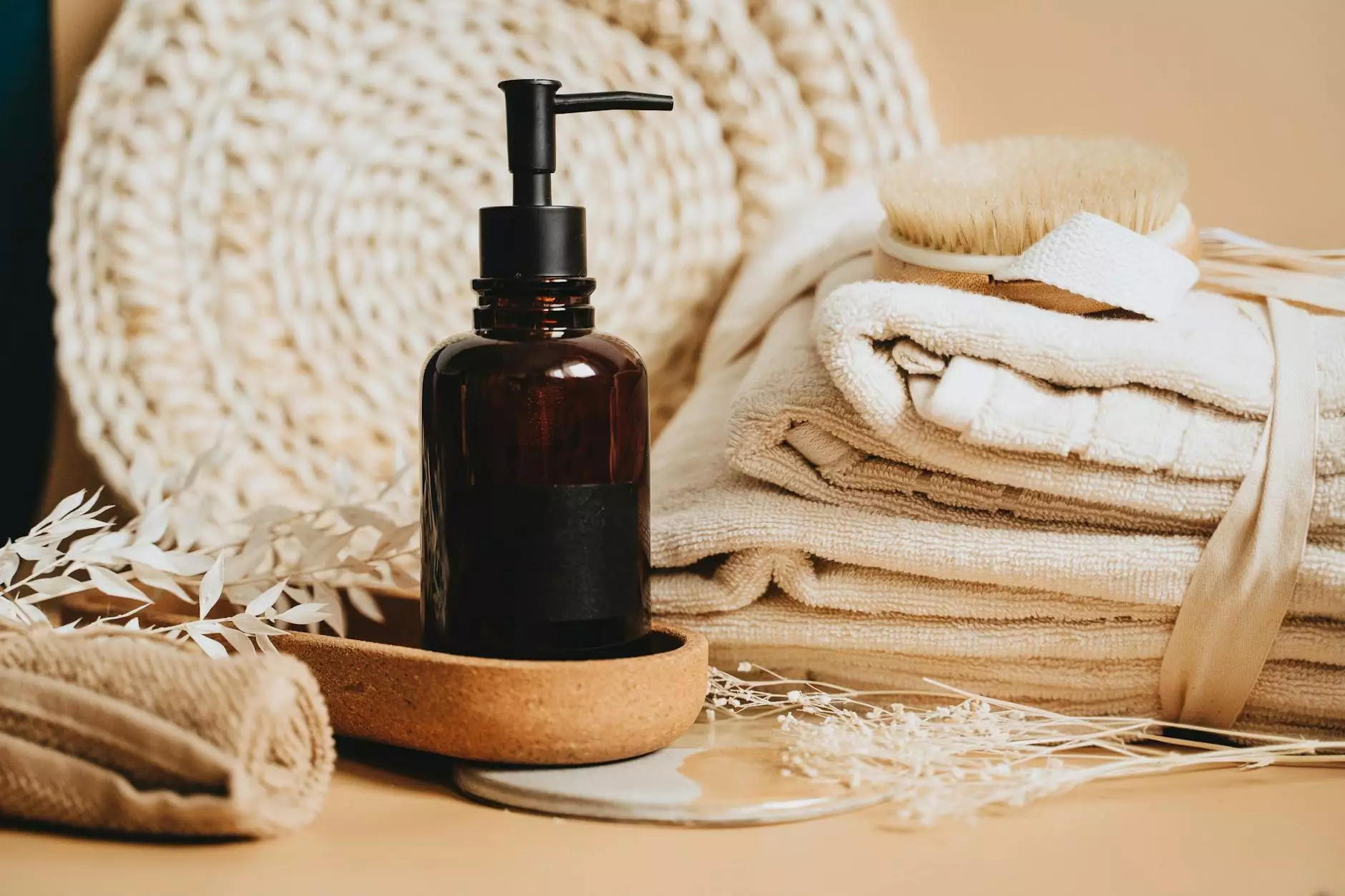
In the rapidly evolving landscape of technology, businesses are continuously seeking innovative ways to improve efficiency and effectiveness. One of the pivotal advancements in this area is the implementation of image labeling tools for object detection, which are becoming increasingly essential for software development projects.
Understanding Image Labeling Tools
Image labeling tools are software that allow users to annotate images by assigning labels to specific objects within them. This process is crucial for training machine learning models, particularly in the field of computer vision. By accurately labeling images, these tools enable algorithms to learn and make predictions about unseen data.
The Significance of Object Detection
Object detection refers to the ability of a computer system to identify and locate objects within an image or video stream. It has a myriad of applications, including:
- Autonomous Vehicles: Object detection plays a critical role in helping vehicles navigate safely by recognizing pedestrians, road signs, and other vehicles.
- Security Surveillance: Identifying suspicious activities or persons in real-time enhances security protocols.
- Healthcare: Detecting abnormalities in medical imaging, such as tumors in X-rays and MRIs, can significantly aid in early diagnosis.
- Retail: Analyzing customer behavior through video feeds can help in inventory management and enhancing customer experiences.
Components of Effective Image Labeling Tools
When selecting an image labeling tool for object detection, several key components should be considered:
1. User-Friendly Interface
A simple and intuitive interface is paramount; it allows users of all skill levels to efficiently label images without extensive training. The easier a tool is to use, the more effectively teams can annotate large datasets.
2. Robust Annotation Features
Effective image labeling tools offer a variety of annotation options, such as:
- Bounding Boxes: These are rectangles drawn around objects to indicate their presence.
- Segmentation: This involves marking the exact pixels that belong to an object for more precise recognition.
- Keypoint Annotation: Useful for images where the position of certain points is essential, such as identifying a person's joints in posture detection.
3. Integration Capabilities
Compatibility with existing systems and software is crucial. An ideal image labeling tool should integrate seamlessly with other software development environments, making the workflow smoother and reducing friction in data handling.
Benefits of Image Labeling Tools for Businesses
The implementation of image labeling tools in business applications yields numerous benefits:
1. Improved Accuracy in Machine Learning Models
High-quality labeled data leads to improved accuracy in training machine learning models. This is critical for tasks requiring precision, such as object detection in dynamic environments.
2. Increased Efficiency
Automating the image labeling process can save a considerable amount of time, allowing teams to focus on more strategic tasks. Tools that utilize AI to assist in labeling can significantly expedite workflow.
3. Enhanced Collaboration
Many modern image labeling tools offer collaborative features that allow multiple users to work on a project simultaneously. This fosters teamwork and accelerates project completion.
How to Choose the Right Image Labeling Tool
With numerous options available, businesses must be strategic in selecting the right tool for their needs. Here are some criteria to consider:
- Scalability: Ensure the tool can handle the volume of images your business requires.
- Cost-Effectiveness: Evaluate subscription models and features to determine which tool offers the best value.
- Support and Documentation: Accessible customer support and well-documented resources are vital for troubleshooting and optimizing usage.
Best Practices for Using Image Labeling Tools
Employing best practices in image labeling can maximize the benefits derived from these tools. Below are some recommendations:
1. Define Clear Labeling Guidelines
Establishing consistent guidelines for how to label images ensures uniformity across the dataset, which is crucial for machine learning accuracy.
2. Regularly Review and Update Labels
As your business evolves, so too might your labeling requirements. Regularly reviewing and updating your datasets is important to maintain relevance and effectiveness in model performance.
3. Utilize Quality Control Measures
Implement quality checks to verify the accuracy of labeled data. This can involve having multiple annotators review challenging images or utilizing automated error-checking features if available.
Case Studies: Successful Implementation of Image Labeling Tools
To illustrate the efficacy of image labeling tools in enhancing object detection capabilities, let’s examine a few case studies from various industries:
Contextual Example 1: Autonomous Vehicle Development
A major automotive company integrated an advanced image labeling tool for object detection to improve their vehicle's navigation system. By accurately labeling thousands of images from various driving environments, they enhanced their vehicles' ability to detect and respond to pedestrians and road signs, significantly improving safety features.
Contextual Example 2: Healthcare Imaging
A healthcare startup utilized image labeling tools to enhance the detection of tumors in mammography images. By creating a labeled dataset that included numerous annotated images, they trained their AI model to identify potential issues with a high degree of accuracy, facilitating early diagnosis and treatment.
Contextual Example 3: Retail Customer Behavior Analysis
A retail company employed image labeling tools to analyze customer movements within their stores. By labeling video feeds to identify customer interactions with products, they could better understand shopping behavior, leading to more informed marketing strategies and improved product placements.
The Future of Image Labeling in Object Detection
As technology advances, the importance of high-quality image labeling tools for object detection will only grow. Innovations such as automated labeling using AI and improved user experiences will shape the next generation of these tools. Businesses that adapt and invest in these technologies will undoubtedly gain a competitive advantage in their respective fields.
Conclusion
In closing, the adoption of image labeling tools is instrumental in propelling businesses toward success in a data-driven world. The ability to accurately label and process images not only enhances object detection capabilities but also translates to improved operational efficiency, better decision-making, and ultimately, increased profitability. As software development continues to intertwine with artificial intelligence and machine learning, the relevance of these tools will only continue to amplify, making them an indispensable asset for any forward-thinking business.
For those looking to integrate advanced image labeling tools in their business workflows, KeyMakr offers a robust solution that caters to your software development needs. Explore what we have to offer today and take your project to the next level.