Ultimate Guide to Labeling Tools for Machine Learning
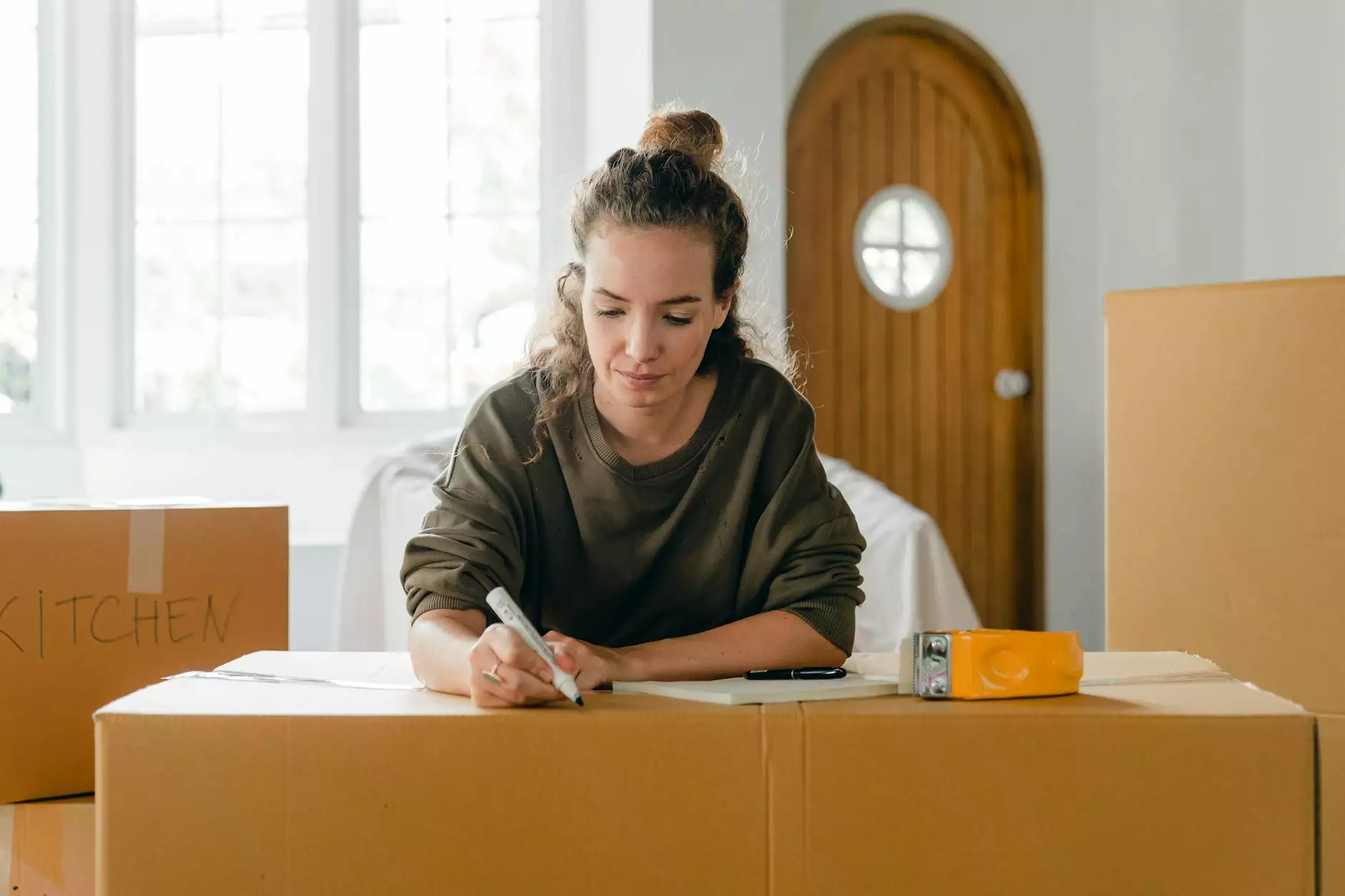
In today's rapidly evolving technological landscape, the importance of machine learning (ML) cannot be overstated. As businesses seek to harness the power of data-driven decision-making, the role of data annotation becomes increasingly critical. Central to this process are labeling tools for machine learning, which facilitate the accurate and efficient tagging of data. In this comprehensive guide, we will delve deep into the significance of labeling tools, explore various types available, and highlight their features and applications, particularly as offered by KeyLabs AI.
The Importance of Data Annotation in Machine Learning
Machine learning algorithms rely heavily on data; however, data in its raw form is often not usable. Data annotation transforms this raw data into a structured format that algorithms can understand and learn from. Here are several reasons why data annotation is essential:
- Training Accurate Models: Without annotated data, machine learning models fail to learn patterns effectively.
- Improving Algorithm Performance: High-quality labeled data results in better accuracy and performance of ML models.
- Customizability: Different types of data (e.g., images, text, audio) require specific labeling approaches, making annotation crucial for tailored solutions.
Understanding Labeling Tools for Machine Learning
Labeling tools for machine learning are software applications designed to assist in the data annotation process. These tools provide functionalities that streamline labeling, ensure consistency, and improve the speed of data preparation. There are several categories to consider:
Types of Labeling Tools
Labeling tools can significantly differ based on their features, usability, and the specific type of data they handle. Here are some primary categories:
- Image Annotation Tools: Platforms designed specifically for image data, allowing users to annotate objects, faces, and scenes within images.
- Text Annotation Tools: These focus on labeling text data, which can include sentiment analysis, named entity recognition, and more.
- Video Annotation Tools: They provide capabilities for frame-by-frame analysis, allowing users to label actions or objects in video sequences.
- Audio Annotation Tools: Designed for audio data, these tools help in transcribing audio into text or labeling specific audio events.
Key Features of Effective Labeling Tools
When evaluating labeling tools for machine learning, several key features should be considered to ensure the chosen platform fulfills your project's requirements:
- User-Friendly Interface: An intuitive interface enables quick onboarding and lessens the learning curve for new users.
- Collaboration Tools: Effective labeling tools facilitate team collaboration, allowing multiple users to work on the same dataset simultaneously.
- Integration Capabilities: The ability to integrate with other tools and platforms is vital for a seamless workflow.
- Quality Control Features: Tools that offer features such as review mechanisms and automated quality checks ensure high labeling standards.
- Scalability: Your labeling tool should be able to scale with your growing dataset and project needs.
Top Labeling Tools for Machine Learning
At KeyLabs AI, we understand the diverse needs of businesses when it comes to data annotation tools. Here are some of the top labeling tools widely recognized in the industry:
1. KeyLabs AI Annotation Platform
Our flagship product, the KeyLabs AI Annotation Platform, is designed to deliver:
- Comprehensive Data Annotation: Supports multiple data types including image, video, text, and audio.
- AI-Powered Automation: Leverages machine learning to assist in label predictions, thus speeding up the annotation process.
- Real-Time Collaboration: Team members can work simultaneously, share insights, and improve productivity.
2. Labelbox
Labelbox is another popular tool, offering a platform that focuses on:
- Customization: Users can create specific labeling workflows tailored to their needs.
- High-Quality Reviews: Features built-in quality control to maintain high annotation standards.
3. Supervisely
Supervisely is well-regarded for its performance in image annotation, particularly in:
- 3D Point Cloud Annotation: A unique feature for those working with 3D data.
- Pre-trained Models: Offers access to various models for specific annotation tasks.
4. Snorkel
Focusing on the power of weak supervision, Snorkel allows users to:
- Label with Less Data: Use existing unstructured data and provide weak supervision signals for effective training.
- Efficiently Manage Annotations: Users can iteratively improve the model based on new insights.
The Role of Automation in Data Labeling
As the demand for labeled data grows, automation becomes increasingly important. Automated labeling tools use machine learning algorithms to perform certain labeling tasks, significantly reducing time and effort involved in manual annotation. Here are some key benefits of using automated labeling:
- Speed: Automated tools can label large volumes of data quickly, allowing ML teams to focus on more strategic tasks.
- Consistency: Automated annotations are less prone to the variability seen in human labeling.
- Cost-Effectiveness: Reducing the need for extensive human annotators lowers overall project costs.
Challenges in Data Annotation
Despite the advantages, several challenges can arise during the data annotation process:
- Data Diversity: Different data types require unique approaches, leading to complexity in choosing the right tool.
- Quality Assurance: Ensuring that labeling is accurate and high quality can be resource-intensive.
- Scalability Issues: As datasets grow, maintaining consistent annotation quality can become challenging.
Choosing the Right Labeling Tool for Your Business
When selecting the most appropriate labeling tools for machine learning, consider these critical factors:
- Dataset Type: Analyze the type of data you are working with and choose tools that specialize in that data type.
- Team Size & Skills: Ensure the platform is suited for the skill levels of your team and encourages collaboration.
- Budget Constraints: Different tools have varied pricing models; choose one that aligns with your budget while delivering quality.
Future Trends in Data Annotation and Labeling Tools
The field of data annotation is constantly evolving, and several trends are emerging that will shape the future of labeling tools for machine learning:
- AI-Augmented Annotation: Expect to see more sophisticated AI-driven annotation tools that not only assist in labeling but also learn from user corrections.
- Crowdsourcing Solutions: Companies may increasingly turn to crowdsourcing to handle large-scale annotation projects, leveraging the workforce of remote contributors.
- Increased Focus on Ethics: The ethical implications of AI and data usage will prompt more discussions on responsible data handling practices.
Conclusion
The world of labeling tools for machine learning is pivotal in refining and enhancing machine learning models. As the amount of data generated continues to grow exponentially, the demand for effective, efficient, and high-quality data annotation solutions becomes ever more pressing. By choosing the right tools and investing in appropriate solutions like those offered by KeyLabs AI, businesses can ensure they harness the full potential of their data, ultimately leading to smarter decisions and improved outcomes.
To explore how KeyLabs AI can help your business achieve its data annotation goals, visit keylabs.ai today.